subscribe to our mailing list:
|
SECTIONS
|
 |
 |
 |
Additional critical
remarks in regard to Witztum, Rips, and Rosenberg's "code"-related
publications
By Mark Perakh
Posted May 8, 1998
CONTENTS
- Introduction
- About
the "proximity" hypothesis and variations in the four statistics' values
- Discussion of
the "proximity" postulate
- The four
aggregate criteria of "proximity"
- Behavior of
cumulative criteria Pi in the same test
- Behavior of
cumulative criteria Pi in different tests
- About
the relationship between WRR's results for one million permutations vs
one hundred million or one billion permutations tests.
- The
real statistics is in distributions
- Conclusion
- Appendix (5
examples illustrating the statements made in this article ).
- Endnote
- References
I intend to cover in this paper several topics, related to
Witztum et al (WRR) claims asserting that the Equidistant Letter
Sequences (ELS) they had found in the Book of Genesis constitute a deliberately
inserted "code." WRR base their claim on a statistical study which,
as they maintain, had demonstrated that pairs of ELS related to each other by
meaning, appear in the Book of Genesis in an unusually "close proximity" to each
other. WRR claimed that their statistical data showed extremely low
values of "general significance" being in some tests as low as
0.000000017, and in other cases also very small, such as 0.0000028 and the like.
In my other articles in this Web site [1,2,3] I have
offered a number of points indicating that WRR's results seemed to contradict
basic rules of Probability Theory and Mathematical Statistics; that the
basic postulate by WRR, accepting the notion that the deliberately inserted ELS
are expected to display "close proximity" has no logical, or factual, or
religious foundation; that their results with the Book of Genesis have
left many questions unanswered, etc. Critical remarks in regard to
WRR's publications, suggested from various viewpoints, and being, in my view,
highly convincing, have been voiced, some in the press, but mostly in Web
postings, by many other writers, such as Dr. B. McKay, Dr. B. Simon,
Dr. G. Kalai, Dr. D. Bar-Natan, A. Gindis, A. Levitan, Dr. J. Price, Dr.
A. Hasofer, Dr. J. Rosenstein, Dr. M. Bar-Hillel, Rabbi M. Schiller, D.E.
Thomas, G. Cohen, and others (see references in [1,2,3]). In this paper
I intend to discuss some topics which so far remained more or less beyond the
dispute, but which, in my view, are important additional elements of the case
against WRR's claims.
These topics are as follows: 1) Discussion of the
foundations of the "proximity" postulate; 2) Discussion of the
significance of the variations in the values of four statistics, suggested by
WRR and denoted in their publications P1, P2,
P3, and P4. 3) Discussion of the data by WRR
showing how the criterion of "close proximity" they used changed when the number
of permutations of their data lists increased from 1 million to 100 million or
to 1 billion.
The original paper by WRR [4] was published in the Statistical Science
journal in 1994. I'll will refer to it as WRR1. Later, WRR offered
additional articles, which have not been so far published in scientific press,
but have been made available in the form of preprints, Web postings, etc. In
particular, one of those articles [5] was titled "Equidistant Letter Sequences
in the Book of Genesis. II Relation to the text." I will refer to it
as WRR2. Another article by WRR [6] which I will refer to as WRR3, was
titled "Hidden Codes in Equidistant Letter Sequences in the Book of Genesis. The
Statistical Significance of the Phenomenon." That article contains the text of
WRR's presentation to the Israeli Academy of Sciences, in 1996. Originally, it
was written in Hebrew, but later became available as a preprint in English
translation.
In the list of references at the end of this article, there are links to
the locations (including postings in this site) where the mentioned three
articles by WRR can be viewed.
In WRR1 Witztum et al introduced four quantities, which they named
statistics P1, P2,
P3, and P4. For each of these four quantities, WRR
suggested a formula. (Actually, WRR provided only two formulas, one for both
P1 and P3, and the other for both P2 and
P4. They applied criteria P3 and P4 to a
data list modified as compared to the list utilized when applying P1
and P2. This distinction is if no consequence for our
discussion of those four criteria). WRR consider these four quantities as
overall statistical measures of "proximity" of ELS pairs in texts under
investigation. The lower is the value of any of these four quantities, the
"better," according to WRR, is the "proximity" of pairs of ELS under study, in
the given text.
We can see that WRR had implicitly suggested several postulates. The first
postulate is that there exists such an objective, measurable property of a text,
which they named "proximity." The very existence of such a measurable objective
property is by no means axiomatic. To verify if such a measure has a real
meaning, a rigorous mathematical model of a text must be first developed. No
such model was ever suggested. It is possible, that no meaningful single-valued
quantity can be defined in a logically uncontroversial manner, which would
reflect the behavior of a text in regard to the "proximity" of any text's
elements to each other.
There are numerous examples of a situation, when a certain integral property
cannot be defined, and therefore any measurements of it are aimless. Some
examples of such situations are discussed in the Appendix to this
article (items 3 and 4 in the Appendix). Indefinable quantities may be of
different nature. Since I am a physicist, it is natural for me to provide an
example from Physics. Another reason to consider the following example is
the similarity of some of its aspects to the situation with ELS in a text.
The example in point is the so called Barkhausen effect. This effect has
been thoroughly studied, analyzed, explained, and utilized as a tool for
investigation of many properties of ferro- and ferrimagnetic materials.
There is a reasonable theoretical model of that effect. Its essence is as
follows. If a ferro- or ferrimagnetic sample is being magnetized in an
external magnetic field, the magnetization of the sample is increasing, along
with the increase of the external magnetizing field. However, even if the
magnetizing field is increasing in a continual way, the magnetization of the
sample is increasing via thousands of small discontinuities
("Barkhausen jumps").
Many features of this effect has been studied, including the distributions of
Barkhausen discontinuities over their duration, and over their amplitude, and
over their shape (on magnetization vs time scale). etc. Much is
known about the mechanism of those "jumps" and about their relationship to many
other properties of the sample, such as demagnetizing factor, saturation
magnetization, remanent magnetization, etc. However, there is no such
integral quantity which might be named "the value" of Barkhausen effect
for a given sample. The difficulty is not mathematical. The physical
nature of the effect is such that no single-valued integral property of a sample
can be defined which would characterize Barkhausen effect in a logically
consistent way.
In a certain sense, the phenomenon of ELS that are present in a text in large
numbers, and are somehow distributed over their lengths, and over their skips,
etc, has certain features in common with Barkhausen "jumps." Of
course, similar does not mean identical. The nature of ELS is quite
different from Barkhausen's jumps, but there is enough of a similarity to guess
that possibly there is also no such an integral single-valued
characteristic of a text as the "proximity" between ELS.
There is a reasonable mathematical description of a ferromagnetic body and of
Barkhausen effect. Even having such a description is not sufficient to
define a quantity to be named "Value of Barkhausen effect." There is no
such mathematical descriptions of a text. Without it, there is no
certainty that it is possible to define, in non-controversial terms, a
"proximity" between any elements of a text, as a single-valued quantity.
Some other examples (one of which, namely example 3 in the Appendix, is
closer to WRR's actual calculations) illustrating a situation where an
integral criterion of a certain property of a conglomerate of elements
cannot be defined, are given in the Appendix to this
article (example 3 and 4 in the Appendix).
Hence, if a quantity named "proximity" is suggested, its very objective
existence is not axiomatic, but has to be postulated. If it happens that such a
quantity is not definable, then different methods developed for its measurement
will most likely produce different, and meaningless results. The
verification of the postulate in question can be performed by considering the
results of that quantity's measurement and judging, by its behavior, if indeed
the measured quantity behaves in a non-contradictory way. (We will try to
judge the behavior of the "proximity" suggested by WRR to see if it behaves in a
reasonable way).
Now, let us follow WRR, and accept the postulate about the existence of a
measurable quantity they named "proximity." The next postulate inevitably to be
formulated, either explicitly, or implicitly, will relate to the quantitative
measure to be chosen for "proximity's" calculation. One thing is to accept the
postulate that "proximity" is an objectively existing property of a text, but
another thing is to define its measurable characteristics. WRR suggested four
aggregate measures of "proximity," P1, P2
, P3, and P4.
The question which arises is, why four different measures?
There can be various reasons for adopting more than one experimental
criterion of a phenomenon. One such reason is often the desire to verify
the measurement's results by two (or more) independent methods. We will
see, though, that this was not the reason behind WRR's choice of four
"statistics." Indeed, in many tests, WRR calculated only 2,
and in a number of tests, only one out of four P's. Their
justification for the choice of this or that of four P's was, as they indicated,
the choice of that P which generated the "best" results. As it can
be seen from WRR's articles, they realized that each of four P's has certain
limitations, and therefore they considered it necessary to derive four measures,
each allegedly for a specific purpose. In the actual use
of P's, however, WRR simply used a P that produced a "better"
outcome of their measurements.
Whatever reason may WRR have for the derivation of four separate measures of
"proximity," if these measures have any objective contents, they
necessarily must produce results that do not contradict each other.
As we will see, the results obtained by using various P, almost without
exception, actually did contradict each other.
Let us look at the following situation. Assume we want to measure a certain
property X of two objects of the same nature, object A and object B.
We are offered two different methods to measure X. When the first
method is used, the values of X turn out to be XA1
for A and XB1 for B. When the
second method is used, we find instead values of X to be XA2 and
XB2 . Analyzing the results of the measurements, we see that the
first method showed that XA1>XB1, but the second method
showed that XA2<XB2. So, using one method, we
found that property X has a larger value for object A than it has for B, while
using the second method, we found that property X has a larger value for object
B than it has for A. These results are mutually exclusive. The unavoidable
conclusion is that either method 1, or method 2, or perhaps both methods are
unreliable. One more possible explanation may be that X is simply not an
objectively existing property of our target objects A and B.
After the first version of this paper was posted, Mr. Alec Gindis (private
communication) suggested that I add a more specific example of the
above described situation. Such more specific example is given in the Appendix to this
article (example 1 in the Appendix).
The above described unfortunate situation is what happened in WRR's tests, as
it is shown in the following two subsections.
For all of the tests described in WRR1, and for many tests described in WRR3,
WRR had calculated values of P for the explored texts, using both the
"correct" list of "appellations/dates" (see the explanation in [3]) and a large
number of its scrambled versions which served as controls. In some of the tests
described in WRR3 (like those tests referred to as "Title sample sets") WRR used
a different method. In those cases, only one "title" expression served for all
permutations. In these tests, the process, briefly, involved permutations
of the letters in one of the words of the "words pairs" under investigation,
while preserving the other word in the pair, namely the so called "title,"
intact. While referring to both permutations methods used by WRR, we will
be using words "data lists" or simply "lists" for both techniques, where
in one situation such "data lists" contained only one "title" expression
vs a multitude of "matching" expressions, while in the other situation
the "data list" contained two groups of "matched" expressions, which could be
mismatched by shuffling one group vs the other).
Naturally, as formula for P1 and P3 differs from that
for P2 and P4, and, also, the structures of data lists for
P1 and P2 are slightly different from those for
P3 and P4, values of P's are also different for
each of P1, P2, P3, and
P4. That is what is expected, of course. Next,
though, WRR place the obtained values of each of four P's in the ascending
orders. They assign to the version of the data list that turned out to have
the minimal value of P, rank 1. The version of the permuted data list
that has the next smallest value of P is assigned rank 2, etc. Somewhere
on the ladder of ranks so created, there is the original, not scrambled
data list. Let's say it has rank r. It means that in the entire
set of tested data lists, there are r-1 scrambled lists, whose
rank is lower than for the "correct," not scrambled list.
What happened in WRR's tests, was that ranks r found for
the "correct" (non-permuted) lists were different for each of four
versions of criterion P. Let us see what is the meaning of that situation.
If on the ladder of ranks corresponding to P1,
the rank of the "correct" list is r1, there are in that
ladder r1-1 scrambled lists with ranks below that for the
"correct" data list. On the other hand, if on the ladder corresponding to
criterion P2, the rank of the "correct"list is r2,
then, according to P2, there are not
r1-1 but
r2-1 scrambled lists with a criterion of proximity P below that
for the "correct" list. Then, if, for example, it has been found that
r1>r2, then obviously there are at least
(r1-1) - (r2-1)=r1-r2 lists which,
according to criterion P1 have "proximity" below that for the
"correct" list, while, according to criterion P2, the same, at
least r1-r2 lists, have "proximity" higher than that
for the "correct" list. The same consideration applies to the different "ranks"
found for P3 and P4.
Consider a numerical example. In Table 8 in WRR3, the value of the
"rank" for P1 is given as 14, while the value of the "rank" for
P2 (for the same sample set, and in the same test) is given as 2723.
Hence, if we believe the value of P1, there are in that sample set
only 13 permuted lists for which the "proximity" is "better" than for the
non-permuted list. However, if we believe instead the value of P2,
then there are, among the explored permutations of the list, not 13, but 2722
versions with the "better" proximity than for the non-permuted list. In other
words, there are, among the explored permutations of the list, at least
2722-13=2709 lists which, according to the values of P1,
have a higher value of "proximity" measure than the original, non-permuted list,
but according to P2, the same, at least 2709, lists have a
lower value of the "proximity " measure than the original, non-permuted
list. The results, obtained by two WRR's methods for those, at least
2709, permutations, are mutually exclusive.
The inevitable conclusion is that either P1, or P2, or
both of them are not objective criteria of the "proximity," or, possibly, that
the "proximity" itself does not exist as an objective property of the
text. It applies also to P3 and P4.
Except for one test only, namely that for the "Nations sample set" (table 5
in WRR3), in all other tests where WRR reported values for more than one of four
P's, the values of "ranks" reported by WRR turned out to be different for each
P.
Therefore, if "proximity" itself, as implicitly postulated by
WRR, is indeed an objective property of the texts, then the only possible
conclusion is that at least two of the four P (for example,
P1 and P3)
or perhaps, all four of them, are not objective measures of that
"proximity."
I believe that the above simple consideration alone renders invalid the
results and conclusions offered by WRR (see more about it in the Appendix, examples
1 through 4).
COMMENT
Admittedly, the above considerations may meet rejection on the part of people
specializing in Mathematical Statistics, since their mindset may well be quite
different from that of a physicist. Indeed, in Mathematical Statistics there is
an established procedure for "hypothesis testing." As one can though find
in any text of Mahematical Statistics, the concept of a general scientific
hypothesis is not the same as the concept of a hypothesis to be tested in
Mathemathical Statistics. For example, the following scientific hypotheses
cannot be subjected to the statistical hypothesis test: a) Hypothesis
that the diameter of Mars is smaller than that of Venus. b) Hypothesis that all
energy on the earth has its origin in the Sun, c) Hypothesis that in a specific
car accident the guilty party was the truck driver who sped across the
intersection, etc. Actually, there is more scientific
hypotheses that cannot be subjected legitimately to a statistical hypothesis
testing than those that may.
A statistical hypothesis necessarily deals with random variables.
Otherwise a hypothesis may not be treated statistically.
This difference between a statistical and a general scientific
hypotheses is conducive to the development of different mindsets among,
say, physicists, on the one hand, and specialists in Math. Statistics, on the
other. As one of the consequences, while physicists may sometimes
underestimate or misinterpret the validity of statistical data, the specialists
in Math. Statistics are naturally inclined to sometimes attribute to a
statistical test more cognitive value than it is warranted by the power of that
test. The results of a statistical test, while often possessing a very strong
cognitive significance, in many other cases may lack it either partially or
completely.
Consider the following trivial example. Assume a study has been
conducted which has proved statistically that there is much fewer cases of
tuberculosis among people owing a golden wristwatch than among people owing no
watch or a watch made of steel. Even if that study has been conducted
impeccably from the standpoint of statistics, showing a very strong correlation
between the ownership of gold watches and rare occurrences of tuberculosis,
obviously it would not at all mean that a gold watch is a good cure for
tuberculosis.
Switching to the language of Math. Statistics, we may assert that rejecting a
null hypothesis in favor of the alternative hypothesis, which
is the legitimate outcome of a statistical test, never means that the
alternative hypothesis is correct. It only means that, within the framework of
the particularly formulated problem, the alternative hypothesis is more likely
than the null hypothesis. Sometimes such a conclusion may have a very
solid cognitive value. Often it may not.
Returning to the case of four statistics P1 , P2
, P3 , and P4 in WRR, I would like
to indicate that, even if the data set chosen by WRR were "correct" (and even
that is highly doubtful) then the low ranks of the identity permutations,
different as they are for different P, can be considered as a sufficient ground
to reject WRR's null hypothesis. As to an altertnative hypothesis, WRR
have never formulated it. It seems though to boil down to their
final statement that "close proximity of related ELS in the Book of Genesis is
not due to chance." And that is precisely where the discrepancies between
the ranks of the identity permutation, obtained through different P, indicate
that the rejection of their null hypothesis by no means signified the acceptance
of their not-formulated alternative hypothesis, other than within the very
narrow limits of a purely statistical evidence, which is though quite
insufficient as a general scientific evidence. Low ranks of the "correct"
list of appellations/dates, which are different for different criteria
P1, P2 , P3
and P4 have no more of a proof value than has
the correlation between gold watches ownership and tuberculosis.
The considerations of subsection c) related to the behavior of the alleged
aggregate criteria of "proximity" P1 , P2 , P3
, and P4 , when all four of them were applied to the same
sample set and in the same test, which of course was of the paramount
significance for the determination of the validity of those criteria. Now let us
see how these alleged measures of "proximity" behave when exploring different
sample sets.
Table 1 in WRR3 provides the "ranks" of the original, non-permuted data list
among 1 million "competitors," for the case of the "2nd Sample set." Let us
denote the rank determined by using Pias r i. The ranks in Table 1 are in the
following ascending order: r4 < r2< r1 < r3. (The lowest rank
of the non-permuted list among 1 million competing permutations, namely 4, was
obtained using criterion P4;
the next lowest rank, namely 5, was obtained by using P2 , the
next one in the ascending order of ranks, namely 453, was obtained
by using P1 , and the highest rank out of four ranks, namely 570, was
obtained using criterion P3).
Now look at table 3 in WRR3, where the results of a test on the "1st sample
set" are given. Now the ascending order of ranks of the non-permuted list among
1 million of "competitors" is as follows: r2 <
r4 < r3 < r1 . It is a completely
different order of r's as compared with that in table 1. For example, while in
the test on the 2nd sample set (table 1) criterion P4 produced the lowest rank out of four measured ranks
for the non-permuted list, and criterion P3 produced the highest rank
for the same non-permuted list, in the test on the 1st sample set
(table 3) the lowest rank was produced by P2 , and the highest rank,
by P1 , etc.
Look now at table 8 in WRR3. It relates to the test conducted on "Title
sample set B". In that table are given only the ranks of the non-permuted list
(among 100 million competitors) obtained by using only two of the four criteria,
namely P1 and P2.
It was found in that test that r1 <
r2. This order of "ranks" is again different, both from those given in Table
1 and Table 3.
Hence not only different P's produce incompatible values of "ranks" in the
same test, as it was shown in subsection c) above, there is also no consistency
whatsoever in the orders of ranks these P's produce in different tests. Any
Pican produce a lower rank
of the non-permuted list than any other Pj
in some test, but in another test the same Pican produce a higher rank than Pj .
Such erratic behavior reinforces the conclusion of
subsection c) and leads to the suggestion that indeed the values not only
of any two of P's but rather of all four of P's are accidental numbers without
any objective contents.
There is simply no way for any measure reflecting any objective
property of anything to behave in such a haphazard, erratic manner.
(The behavior of P's described in the above two subsections hints at a
possible deeper fault of the WRR's procedure than just the unreliability of four
P's themselves. Namely, the "metric" ("c-value,") which was the starting
point for the calculation of all four "statistics," was apparently defined by
WRR in an unnatural way, not reflecting a real meaningful "distance" between
ELS. Indeed, as A. Hasofer [7] has shown, it is easy to construct
examples where c-value will produce "large" distances for ELS that are obviously
close to each other, and "short" distances for the ELS that are obviously
located remotely from each other).
Hence, just by viewing the behavior of the "proximity" measures they
suggested, WRR had to derive the only one possible conclusion, namely that there
was something wrong either with their experiments or with their
interpretation of the observed data. Unfortunately, WRR chose to except
the obviously doubtful results as scientifically sound. That is not how a
scientific research is supposed to be conducted.
In WRR3 [6], which, as mentioned before, is the thesis of
WRR's presentation to the Israeli Academy of Sciences in 1966, these authors
reported on additional experiments performed after WRR1 was published.
Most of the material in WRR3 repeats WRR1. There are a few new elements,
though, in WRR3 as compared with WRR1. One such element is the addition of
results of experiments conducted by H. Gans, who used the same technique as in
WRR1 but this time exploring a possible "code" connecting the Rabbis names
not with their dates of birth/deaths, but with locations they were born or
died in. Another additional set of tests was conducted by WRR in which the
names of 68 nations derived from the names of Noah's descendents, were matched
to four "characteristics" of these nations (for example, its language). These
additions did not add anything principally new to the previous results reported
by WRR, as they were based on exactly the same technique and applied to the same
basic text.
Another new element in WRR3 as compared with WRR1 was the
extension of their measurements from one million permutations to one hundred
million of permutations, and, at least in one case, to one billion of
permutations. Surveying the material gathered in WRR3 shows some
remarkable features in their tables of experimental data.
Before discussing the particular data in WRR3, let us make some
very simple calculations. As mentioned before, WRR provide in their tables
the values of what they call "ranks" of the "correct data" lists,
where data lists in some tests were appellations of famous rabbis'
vs their dates of births or deaths, while in some other tests, reported
in WRR3, the data list comprised, for example, names of the Rabbi's
vs names of the locations where the Rabbis were born or died, and
the like. If the "correct" list had rank r, it means there were found
g=r-1 scrambled lists whose "proximity" criterion P was found to be smaller than
for the "correct" list.
"Rank" is not an extensive quantity, as it is simply the
serial number of a certain permutation on an arbitrarily chosen scale, where the
values of P are placed in an ascending order, and for each P in the ladder so
created, a real natural number is assigned in the ascending order of natural
numbers. Since "rank" is not an extensive quantity, its mean value has no
material meaning.
Let us denote the total number of explored permuted lists as
N. For example, in WRR's articles,
N was in some cases 1 million, in some other cases 100
million, and, at least in one case, 1 billion. Let us consider the overall set
of N permutations as the sum of
n subsets, each subset comprising
m permutations. For example, if
N is 100 million, then we consider it being the sum of
100 subsets (n=100) each comprising 1 million
(m=1,000,000) permutations. If in any particular
subset of permutations, whose serial number is i, it
was found that the rank of the non-permuted text
wasri, then obviously gi=ri-1. Unlike rank
r, quantity g is an
extensive one, and the sum of its values has a very simple meaning. If
n subsets of tests have been performed, and in each of
them the value of giwas found, then for the entire set
of N permutations, the value of g
(we denote it g*) can be found simply by
summation of all gi:

Since ri =gi+1, obviously the
rank r* of the non-permuted text in the entire set of
N permutations, which set consists of
n subsets, is
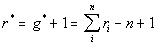
Using this simple formula, we can easily find what is the
rank of the non-permuted list in the entire set of
N permutations, if we know values of
ranks of that list in each of
n subsets of permutations.
WRR never provided the information derived from all
n subsets of permutations. In WRR3 there are though two
cases when the information is available for both the set of
N permutations, and one subset
of m permutations.
Let us look at these results.
Table 1 in WRR3 provides the values of all four P's for the "2nd
sample set." The minimum value of rank of the
non-permuted list among the four P happened to be for P4 and equaled 4.
This rank of the non-permuted list
of appellations/dates was obtained in the subset of 1 million permutations.
Table 2 provides the results of an analogous test, but this time with 100
million permutations. True to their practice of choosing the "best" outcome, WRR
made measurements, in the larger sampling, for only one of the P's, namely for
P4 , and the
corresponding rank was found to be 59.
Since the entire set of 100 million permutations consists of
n=100 subsets , in each of 1 million permutations-long
subsets the mean value of g over one subset is
g m = g*/100= (r*-1)/100=
=(59-1)/100=0.58.
If the mean value of g per one subset of 1
million permutations is 0.58, what is the probability that in an arbitrary
subset the value of g will happen to be 3 (which
corresponds to the rank of 4 reported in table 1)?
We can estimate the probability in question by assuming that in the 32!
combinations of all possible permutations of the data lists, the values of
g are distributed following Poisson distribution [8].
Then, as it was actually calculated by Dr. B. McKay (private communication) the
probability of g in an arbitrarily chosen subset to be
3 is between 0.02 and 0.03. Of course, even though this probability is rather
small, it is not exceedingly so. However, this is just the beginning of the
story.
Looking at the rest of the tables in WRR3, we notice, that besides the above
described tables 1 and 2, there is only one more case for which WRR provided
data both for the entire set of N permutations, and for a subset of it. These
are tables 5 and 6 containing the data for the "Nations sample set." In
table 5 the rank of the non-permuted list among 1
million permutations was found to be 1, both for P1
and P2. This is a result which is rather exceptional among all the results
obtained by WRR, as well as by H. Gans, by B. McKay, etc.
Rank of 1 was almost never observed in all the
multitude of experiments described so far. In table 6, for the same sample set,
but for 1 billion of permutations, the rank, reported
only for P2 , is
17, which is also the "best" of all the
results reported so far. Now, of course, if the rank
among 1 billion permutations is 17, then the occurrence of
rank of 1 in a subset of one million permutations is
not contradictory. The question is, however, how reliable are these
exceptionally low ranks for the "Nations" sample set,
presented in tables 5 and 6 in WRR?
A convincing answer to that question provides an article by D. Bar-Natan, B.
McKay, and S. Sternberg [9]. These three authors have thoroughly analyzed the
"Nations" experiment" by WRR and have demonstrated that the results in question
are highly unreliable. This leaves only one way for us, which is to dismiss the
astoundingly "good" results of the "Nations" experiment.
Then let us look at the rest of the data presented in WRR3. For some
sample sets WRR used 1 million permutations only. For others, 100 million
permutations only. They did not provide any explanations as to why they chose
different numbers of permutations for different sample sets. A natural
assumption in this situation is, especially given the inclination of WRR to
choose for presentation the "best" P, that their decision to choose this or
that number of permutations was also somehow influenced by the desire to present
the best results only.
Let us look, for example, again at table 8 in WRR3. It contains the data for
100 million permutations for what they referred to as "Title type, B sample
set." As mentioned earlier, in that table values of
ranks are 14 for P1, and 2723 for P2. Of course,
when calculating the "significance level" WRR ignored the larger number, and
used the rank of 14. If in one hundred million
permutations the rank was 14, it means the mean value of g was gm
=(r*-1)/100=0.13. In order for the mean g to be 0.13 in the totality of 100
subsets, its value in most of those 100 subsets must have been 0, with only a
few subsets with g=1 or larger. In other words, the value of the
rank in most of the 1 million-long subsets must
be 1, and only in a few subsets it may be 2 or more. Except for the
"Nations" sample set, which has been proved unreliable, WRR have never reported
such low ranks for any sample sets.
Similar situation is with, for example, table 7, which provides data for
"Title type, A" sample set. The rank of the
non-permuted list in 100 million permutations was reported to be 24. It means
the mean value of g in each of the one million-long
subsets must have been 0.23. Again, it requires that the
ranks in most of the one-million-long subsets have values of 1,
and only in a very few of them more than 1. It again is not a common situation
as it was observed in WRR's other experiments.
Similarly "good" are the data in table 10, where the "Title type, D" sample
set is reported. In that case, the minimum value of
rank for P is only 11 out of one hundred million
permutations. It means the mean value of g=0.1, and
the formal "mean" of the rank r to be 1.1. To
get such result for 100 million permutations, one needs to find the
rank to be 1 in the overwhelming majority of the one
million permutations-long subsets. Except for the discredited "Nations"
sample set, WRR never observed such values of ranks when exploring one million
permutations-long sets.
Therefore the results reported in WRR3 look strange. Each time WRR
increased the number of permutations from 1 million to 100 million, or to one
billion, their results improved. One million, one hundred million, or even one
billion all are just tiny fractions of the total number of possible permutations
of the data list which was 32!. The difference between one milion and one
billion is 3 orders of magnitude. On the other hand, even one billion is
smaller than 32! by more than 26 orders of magnitude. Therefore switching
from one million of permutations to one hundred million, or even to one billion
of permutations hardly changed the fact that the used permutations still
constituted a randomly selected very small subset of
the total set of possible permutations. Hence, we could expect that the "rank"
of the non-permuted list would vary between subsets of one million and of one
hundred million, or even of one billion permutations, in a random fashion,
rather than to display a tendency to a measurable improvement of results with
the increase in the number of permutations explored.
If, say, in each case the probability of the reported
numbers to happen by chance was the same 0.02 to 0.03, as in the case of tables
5 and 6, then the probability of all of those results to happen as a
combination, by chance, equals the product of all those 0.03's. For
example, if the results in the four tables shown in WRR3 are taken into
account, the probability of all shown results, in their combination, to
happen can be roughly estimated as about 0.00000008. (Of course,
this number has no real significance, but this estimate shows how very small
values of "probabilities" could be arrived at. Likewise, the very small
"significance levels" produced by WRR are not really of a substantial cognitive
value).
Hence, there was a strange systematic
improvement of ranks reported by WRR when they
increased the number of permutations, the probability of this
systematic increase being very small.
The explanation which comes to mind is, that, in agreement with
the considerations of the previous section of this article,
quantities P1 , P2 , P3 , and P4,
suggested by WRR as alleged measures of "proximity" of conceptually related ELS
in the book of Genesis, actually are not objective measures of some objectively
existing quantity.
If the aggregate
"statistics" used by WRR under the names of P's are not reflecting any objective
property of texts, what criteria can be suggested instead? To answer this
question, let us go back to the example given at the beginning of this article,
namely the example of Barkhausen effect. As mentioned earlier, Barkhausen
effect has been thoroughly studied, understood, and utilized to unearth many
subtle features of the behavior of ferro- or ferrimagnetic samples. One
common feature Barkhausen effect has with ELS in texts, is that both are
conglomerates of many elements. In the case of Barkhausen effect these
elements are Barkhausen discontinuities (magnetization "jumps") while in the
case of texts these elements are pairs of conceptually related ELS.
However, the study
of Barkhausen effect proceeded on a path very different from that chosen by WRR
and by some other people for studying ELS. To investigate ELS pairs, WRR
as well as some other people, who followed WRR in that approach, chose to
utilize cumulative measures, exemplified by "statistics"
P1, P2, P3,
and P4. On the other hand, the
scientists who investigated Barkhausen effect, concentrated mainly on studying
distributions of Barkhausen
"jumps" over their duration, over their amplitude etc. Of course,
Barkhausen effect is just one example of such an approach. Physicists
usually are aware of the limited value of the integral, cumulative measures,
and, whenever possible, try to unearth the
distributions of any effect's elements over its
characteristics. The distributions are much more informative.
Real statistics is in the distributions.
(For those
mathematically inclined, here is a simple example from calculus. If an
integrand expression is known, as well as the limits of integration, the value
of the integral is defined in an unambiguous way. On the other hand, if a
value of an integral is known, it does not reveal by itself what kind of an
integrand expression is responsible for that value of the integral. The
same value of an integral can be due to many different functions as
integrands. In particular, if a distribution function is known, there is
normally only one, quite definite value of its integral at given integration
limits.The opposite statement is not true).
Choosing
cumulative measures, be it P1, P2
etc, or any other similar quantities, means sacrificing the scope of information
about the object, in this case a text, for the sake of simplification.
Therefore, even if P1, P2 etc were
replaced with some other, better chosen cumulative quantities, there is little
hope it would provide a reasonable proof of either the presence or of the
absence of a "code" in a text.
I am not a
computer programmer, but it certainly could be possible to develop a program
capable of analyzing the distributions of ELS pairs over the ELS' lengths,
skips, "distances" between them, etc, plus a concomitant analysis of
their spacewise distribution in the text ("mapping" the text in regard to ELS
locations).
Since skip's and
word's lengths are unambiguous concepts, no problem should arise with
interpreting the distributions of ELS over the words' and skips' lengths. On the
other hand, distributions over "distance" between conceptually related ELS would
be more problematic because of the uncertainty in the "distance"
definition.
One possible way
to circumvent that problem could be to account for the fact that much of the
uncertainty in the "distance" between ELS is contributed by the variations in
the skips' lengths and words' lengths. For a subset of ELS all having the same
skip and the same word's length, the definition of the "distance" would become
much easier to choose. Then, rather than studying one, overall distribution
which would encompass ELS' with all possible words' and skips' lengths, several
separate distributions over the "distance" between the conceptually related ELS
should be studied. Each such separate distribution would be determined for
a "bin" containing ELS with only a specified value of skip and a specified word
length.
An example of a
situation where a cumulative measure provides for a meaningless and misleading
conclusion while a study of distributions sheds light on the actual phenomenon,
is given in the Appendix (example 5
in the Appendix).
Possibly, the
described combination of distributions, including the
"map" of ELS, would reveal certain patterns, specific for various texts.
If it were the case, an argument in favor of a "code" could be then an
indisputable uniqueness of the pattern in question in
the Bible, as compared to all other texts. In other words, such a
hypothetical unique pattern must disappear if the Bible text is randomized (for
example, permuted in whichever way) and also no such pattern must be found in
any real texts other than that of the Bible (possibly only of some specific part
of the Bible). Of course, to perform such a study would be quite time consuming
and tedious. While I would not want to make predictions, my own feeling is
that the result most likely would be still inconclusive, since uniqueness of a
specific text in regard to distribution of various characteristics may occur
naturally by many mechanisms, and not necessarily proves a deliberate
design. However, without such a study, WRR's claims about the existence of
a "code" in the Torah, on the base of calculating some meaningless
cumulative quantities, are even more contrary to the accepted scientific
procedure.
Comment. Since the initial version of
this article had been posted, a new information has become available, proving
again, that when its time comes, a similar idea occurs simultaneously and
independently to a number of people. (All the information I am referring
to in this comment has been obtained via personnal
communications).
a) Dr. R.
Haralick, apparently being dissatisfied with the prospects of solving the
controversy about the "code" by means of continuing experiments employing
criteria similar to WRR's four "statistics," suggested that some other
characteristics of a text have to be explored. Such characteristics would
be identified in the text of the Bible and then tested to see if they disappear
when the text is randomized. (Dr. R. Haralick usually refers to randomized
texts as "monkey" texts). Analogous tests would be performed with
non-Biblical texts. This would enable the researchers to determine if
certain characteristics are unique for the Bible text. Dr. R. Haralick
suggested two possible candidates for the characteristics to be explored, namely
1) Word frequency, and 2)Word clumping. It is easy to see that
Dr. Haralick's idea jibes well with my suggestion in regard to studying the
distributions of ELS over their parameters, the difference being in the choice
of texts' characteristics to be studied (Dr. R. Haralick invited everybody to
suggest other possible characteristics to investigate; he apparently had no
knowledge yet about my proposal about ELS distributions). Of course, many
problems remain if Dr. Haralick's proposal is accepted for a real experiment.
These problem relate both to a proper choice of the suitable text's
characteristics and to the interpretation of the results. Moreover, the
chosen characteristic must not only be suitable in principle, it must also be
relatively easy to measure.
The ELS
distributions over their parameters, suggested above, do not have an inherent
evidentiary advantage as compared with any other possiblle characteristics of
texts. Since, however, until now, the discussion, for obvious reasons, revolved
around ELS, it gives the ELS distributions, within the framework of the
ongoing dispute, a certain special place among all properties of texts.
Studying the ELS distributions seems to be the easiest way to connect the
outcomes of such experiments to WRR's results, which may be not the case if some
other characteristics of texts are chosen for exploration.
(Also,"mapping" the text in regard to ELS spatial distribution, as suggested
above, seems to be a wider concept than just determining word "clumping," as the
"map" would include any evidence of "clumping" as a part of the overall picture
of words' spatial distribution).
b) Dr. B.
McKay went further, having not just suggested to explore various peculiarities
of meaningful text in comparison with their randomized versions, but has
actually performed an extensive series of ingenious experiments in this
direction. Among the features Dr. B. McKay studied are the
following:
1. Correlation
between various letters situated in a close proximity to each other. (For
example, in English letter q is very often followed by u,
etc). 2. Non-even distribution of letters across the entire text. 3.
Non-even distribution of letters within the sentences; 4. A correlation between
letters occupying certain positions in one word and letters occupying the same
position, or different, but fixed, position, in another, closely situated word
(for example between the first letter in one word and the first letter in
another word, or between the first letter in one word, and the last letter in
another word, etc). 5. Variations in letters frequencies between left and right
halves of verses in the Bible (and a similar phenomenon in non-Biblical texts)
as compared with randomized "texts" etc.
In all the above
situations Dr. McKay found strong effects in the meaningful texts, which
disappeared in randomized texts. The phenomena were similar in both the Books of
the Bible and in non-Biblical texts. (Since all the above features of meaningful
texts contribute to the entropies of texts, these finds are in a good agreement
with the hypothesis about the possible role of texts' entropy in making WRR's
"proximity" values nearly extreme in the actual Genesis text as compared with
control texts - see [3]).
As Dr. McKay
indicated, while all the effects he discovered must be connected in a certain
way to the ELS behavior, the exact manner of such connections is hard to figure
out. In view of this, the study of the distributions of ELS over their
parameters, while not being inherently a stronger evidence either for or against
the "code" than any other features of texts, would have an advantage of
being more directly reflecting on the ELS behavior, which has, so far, been at
the core of the "code" controversy.
The above considerations lead to the following
conclusions:
1. The postulate implicitly introduced by WRR in regard to
the objective existence of a property of texts they named "proximity" found no
confirmation in the results of the experiments reported by WRR.
3. The above two conclusions are in agreement with
the other arguments against the claims by WRR, offered in the other articles in
this Web site.
4. A better way to study the phenomenon of conceptually
related ELS would be the investigation of their distributions over various
parameters rather than the use of cumulative measures.
Whereas there is no proof availavle that there are no
"codes" in the Bible, the alleged proofs suggested so far in favor of the
hypothesis of the "code's" existence, do not meet a number of necessary
requirements to be accepted as real. Until (and if) such rigorous proofs
are offered, the most reasonable explanation of the data reported by WRR remains
the suggestion that the phenomenon is due to random coincidences of ELS.
Example 1. The case of two faulty measuring
devices
As it was indicated in the body of this article, the following
example is provided here at the request by Mr. Alec Gindis, as a more specific
illustration of the situation when two measures of the same phenomenon supply
mutually exclusive results.
Imagine that an American by the name of John went to
Europe to visit a friend in Germany, and took with him his Buick. His friend,
whose name was Franz, owned an European car, an Audi. They set out on a
trip in two cars, whose first leg was from Stutgart to Munich.
Buick's odometer was, naturally, graduated in miles, while Audi's odometer was
in kilometers. When they arrived in Munich, John read his odometer and
found that the distance from Stutgart to Munich was, say, 120 miles.
Franz, though, claimed that they traveled 220 kilometers. They realized, of
course, that the reason for the two different numbers was simply the utilization
of two different scales in their cars. Even though they had no proof that either
of the readings was correct, there was also no reason to doubt the
readings, as the difference between them was expected, whereas they did not
remember the ratio of a mile to a kilometer. Then, though, they continued
their trip from Munich to Nuremberg. When they arrived in Nuremberg, John
read on his odometer that the distance from Munich to Nuremberg was 107 miles,
while Franz read on his odometer that the distance in question was 225
kilometers. Now John and Franz noticed that the two measurements were
incompatible. According to Buick's odometer, the distance from Stutgart to
Munich (120 miles) was larger than the distance
from Munich to Nuremberg (107 miles). According to Audi's odometer,
though, the distance from Stutgart to Munich (220 kilometers) was
shorter than from Munich to Nuremberg (225 kilometers).
Obviously, the two measurements could not be both correct. Objectively,
either the distance from Stutgart to Munich is larger, or that from Munich to
Nuremberg is larger (as we know for sure that these two distances are not
equal). Obviously, at least one of the odometers must be out of order.
Since both John and Franz were patriots, John insisted that Audi's odometer was
wrong, while Franz was confident that Buick's odometer was to blame. They
went to a mechanic who tested both odometers and announced that actually both
devices were unreliable, having thus saved the friendship between USA and
Germany.
The above example may serve as an illustration designed
to clarify the critical comments in regard to WRR's method. This example
necessarily involves a certain simplification. More detailed example, which are
closer to WRR's actual procedure with the text of Genesis, are given in the
following sections of this Appendix.
Example 2. The case of contradictory aggregate measures of a
phenomenon
Let us imagine we decided to compare two countries, such as, for
example, Canada and Mexico, from the viewpoint of the "proximity" of
cities in these countries. Since there are too many cities in each country,
making the task of calculating the "proximity" exceedingly time-consuming, we
decide to limit ourselves to a certain type of cities, for example, accounting
only for the cities with populations of more than 100,000 people. Of course, the
threshold of 100,000 is arbitrary, and choosing another threshold could change
considerably the outcome of our study.
Next we have to define the "distance" between any two cities. We see at once,
that "distance" is an ambiguous concept, as cities are not points on the map.
Each city occupies an area, which varies from city to city both in size and
shape. We try first to define the "distance" between two cities, as, for
example, the distance between the entrances to the city halls of both cities. We
discover soon, that the chosen definition is far from being perfect. For
example, imagine two cities, 1 and 2, that are stretched as narrow strips along
a river. The "endpoint" of the remotest outskirts of city 1 is 60 miles from the
nearest to it endpoint of the outskirts of city 2. However, the distance between
the entrances to the city halls is, say, 100 miles. On the other hand, there is
another pair of cities, 3 and 4, both occupying areas of more or less round
shape. The distance between the remotest outskirts of city 3 and the nearest to
it outskirts of city 4, is 65 miles, which is larger than for cities 1 and 2,
while the distance between the entrances to the city halls of cities 3 and 4 is
75 miles, which is less than for cities 1 and 2. Obviously, the chosen measure
of the inter-city distance, namely between the city halls, fails the test of a
simple logic. Neither the distance between the "endpoints" of outskirts is
logically satisfactory. For people living near that endpoint of city 1 where the
straight road starts toward city 2, city 2 is quite close, but for the people
living at the opposite end of city 1, the distance to city 2 is quite large.
These example shows that the very concept of a "distance" between cities is not
quite obvious and simple, and the definition of a "distance" is a matter of
choice. That choice, which can be made in many different ways, strongly effects
the outcome of the calculation of "proximity."
So far, we had already to make two choices, one being which cities to include
into our investigation, and the other how to define the "distance" between any
two cities. Actually, we have no a priori proof that "proximity" of
cities in a country is an objectively existing property of those countries and
can be defined in a non-controversial and single-valued manner.
Comments:
a) In the case of a text, where the "proximity" of ELS was to be
measured, WRR had to make a number of similar arbitrary choices.
They chose which ELS to account for and which to ignore. They limited
themselves, first, to only what they named "noteworthy" ELS chosen according to
the criterion of what they called "domain of minimality" [4]. Second, they
limited the ELS to be studied to only those ELS which had a skip length below a
certain arbitrarily chosen value, so that the word in question would have not
more than 10 of such ELS in the text. Finally, they limited their study only to
the words containing between 5 and 8 characters. The reasons for those choices,
as they have been given in [4], had little to do with the objective contents of
the "proximity" concept itself. Then, they introduced a very complex
definition of a "distance" between two ELS, which was only one of many possible
choices, and which in many instances ran against logic and common sense [7]).
b) As any analogy, our analogy is not complete or perfect. In the
case of cities in a country, there may be suggested a rather simple, although
far from perfect, way to measure the overall "proximity" of cities by
replacing it with another, related measure, that can be defined in an almost
unambiguous way, namely as the ratio R of the sum of areas occupied by all the
cities in the country, to the total area of that country. The larger is R,
the "denser" are situated the cities in that country, hence the "closer" are,
overall, the cities of that country to each other. (Of course, the area
occupied by a city can be also defined in several different ways. If we
agree to allow a certain level of imprecision, it is possible, though, to agree
on some criterion as to which areas to include into the cities and which to
leave out of consideration).
The described measure R has the advantage of being simple.
It has, though, many drawbacks as well. Some of these drawbacks stem from
ignoring the role of the absolute size of a country. Indeed, assume that one of
the two countries has an overall area ten times as large as the other
country. Let's assume that the criterion of "proximity" R, chosen as
described above, was found to be about the same for both countries.
Obviously, for the two countries in point, this criterion is meaningless.
Indeed, let us say, in both countries the area occupied by the cities is 1/3 of
the overall area of the country. Then 2/3 of each country's area is "free"
from cities. Obviously, in the larger country this "free" area is ten
times larger than in the smaller country, and, hence, the distances between the
cities are much larger than in the smaller country despite of the equal values
of the "criterion" R we chose. Our criterion R implicitly assumed that
both countries were of about the same size.
Other drawbacks of the criterion R of "proximity," chosen as
described, stem from the fact that calculating this criterion involved an
"averaging" procedure, and averaging quite commonly hides many important
features of a phenomenon [11]. To illustrate this point, consider two
countries of about the same size, for which also the criteria R of "proximity,"
chosen as described above, were found to have the same value. Let us
assume that in one of the two countries, 90% of the cities are concentrated
along a sea shore, within an area which constitutes 10% of the overall area of
the country, the rest being uninhabitable desert or mountains. In the
other country, though, its cities are distributed almost evenly over the
country's territory. Obviously, in this case the equal values of the
"proximity" criterion R, chosen as described, are of little significance, as in
the first country the distances between the cities are much shorter than in the
second country. Our criterion R implicitly assumed similar distributions
of cities over the countries territories, and when these distributions differ,
the described criterion R of "proximity" has very little meaning. Hence,
even in a much simpler problem, namely that with cities in a country, the task
of defining a meaningful criterion of "proximity" is far from being
trivial.
The situation with conceptually related ELS in a text is much
worse. Here, an attempt to employ even the imperfect criterion R,
described above for the case of cities, would encounter much more serious
difficulties. The "area" occupied by an ELS is a much more ambiguous
concept than that occupied by a city. Also, whereas all cities in a country are
objects of the same nature, in the case of ELS the pairs of ELS related by
meaning have to be singled out to measure their "proximity." Hence,
to define a single-valued criterion of "proximity" between related ELS is quite
a complex task. The results of any choice made cannot be predicted in
advance. The choice of a measure of "proximity" can be justified or rejected
only by testing the results of its utilization. (This is one more example
demonstrating that analogies (even if properly chosen) may be useful for
illustration purposes but have no power of proof).
Let us now go back to our example with cities. Even though the situation with
the cities in a country is easier to handle than the case of conceptually
related ELS in a text, we will, for the sake of an analogy, discuss an
example similar to the situation with ELS in a text. To this end we will have to
ignore the possibility of choosing the ratio R of areas, as described
above, for the estimation of the overall "proximity" of cities, since such
a measure can hardly be used for conceptually related ELS. Consider then other
ways to estimate the overall "proximity" of cities, ways which can be used also
in the case of ELS.
Having chosen a certain definition of the "distance" between two cities, we
have now to choose how to estimate the overall "proximity" of the entire
multitude of cities. Again, we have here many possible choices. For example, we
can choose, as an integral measure of the "proximity," the mean value of the
"distance" between all pairs of cities. Alternatively, we can choose for such a
measure, for example, the product of all "distances," or any other of many
possible combinations of the individual distances between pairs of cities. Since
we may feel that there were ambiguous points in the preceding stages of our
study, we decide to define more than one measure of the overall "proximity." Let
us denote them P1and P2. We expect, of course the value of
P1 to be different from that of P2. For example, the mean
"distance" between pairs of cities and the product of all "distances" will
necessarily be two different numbers. Our goal, though, is not to find certain
numbers for the "proximity" in each of the two countries but to find out in
which of the two countries the cities are situated "closer" to each other. To do
so, we calculate P1 and P2 for both countries and then
"rank" them, assigning rank of 1 to the country that has a lower value of P, and
rank 2 to the other country.
Let us assume that P1 for Canada turns out to be 0.03 while
P1 for Mexico is 0.02. Then, if we rely on P1, we assign
rank 1 to Mexico, and rank 2 to Canada. On the other hand, assume that
P2 turns out to be 0.04 for Mexico and 0.01 for Canada. Hence,
according to P2, we have to assign rank of 1 to Canada, and rank of 2
to Mexico. In other words, if we believe one of our overall measures, say
P1, we conclude that the cities in Mexico are situated closer to each
other than in Canada. If, though, we decide to believe P2, the
opposite conclusion is to be made. These two conclusions are mutually exclusive,
they hopelessly contradict each other. At least one of them must be wrong. Then
we have no choice but to conclude that either P1 or P2,
or, maybe both P1 and P2 are not objective measures of the
"proximity" between cities.
There can be several reasons for P1 and P2's failure to
reflect an objective property of the countries. One reason can be the improper
choice of P1 and/or P2 themselves. Another reason can be
the improper choice of the definition of the "distance" between any two cities.
One more reason can be that the concept of "proximity" as we have
defined it, as an integral, single-valued property of a country, has no real
objective contents.
The contradictory outcomes of the application of the two measures in
the same test are of a crucial significance, negating
any supposedly objective meaning of these measures P1 and
P2. (A similar example can be built illustrating the erratic
behavior of the four criteria P1- P4 in
different tests, by considering a comparison of the cities
"proximities" not betwen just two, but among, say, three or more
countries).
The results reported by WRR are analogous to what was described in the above
example, as it was demonstrated in the body of this article. The only possible
interpretation of the results reported by WRR is that the choice of
P1, P2, etc, for estimating the "proximity" was
unsuccessful, as these P's do not seem to be objective measures of any
objectively existing property of texts. Therefore, all the results reported so
far by WRR in regard to the ranks of permutations of their data lists are
meaningless.
Example 3. One more case of contradictory integral
measures of a
phenomenon
Let us assume we want to compare men in
various countries to judge in which countries men are bigger and in which
countries men are smaller than in Ourcountry. As soon as we start
designing a method to perform our task, we realize that there is no universally
accepted concept of "bigness." We have to introduce one. There are universally
agreed upon concepts of, for example, height, weight, shoulder width, foot size,
arm length, etc, etc. We have to define "bigness" on the base of those common
concepts.
Let us say our first try is to choose height and weight as two measures
of "bigness." Then we have to postulate two relationships, one between height
and "bigness", and the other between weight and "bigness." The simplest
(but not the only one possible) way to do it is to introduce linear dependencies
as follows: Bh=KhH and Bw=KwW, where
H is height of a man, W is his weight, while Kh and Kw are
calibration constants to be defined when we choose methods of measurement of
height and weight. Bh and Bw are two values of "bigness,"
one determined through height and the other through weight of a man. Let us omit
the discussion of units to be chosen for "bigness" because ultimately we will
anyway use ranks of countries rather than absolute values of "bigness."
The two measures of "bigness" do not need to equal each other. What they need to
be, is to be compatible. It means that if man X is "bigger" than man Y according
to measure Bh, he must be also bigger according to measure
Bw.
We start our study with measurements of individual men in various
countries. Let us assume we encounter a situation when there is man X whose
Bh is larger than for man Y, but whose Bw is smaller than
for Y. Who of these two men is bigger? Our test provides no definite answer to
this question. Our conclusion is that, at least for these two men, the concept
of "bigness" as we defined it, is ambiguous. Hence, with respect to pairs of
individual men, the concept of bigness as we defined it is meaningless. At this
stage we don't know if the concept of bigness has any objective contents, i.e if
there is a logically consistent way to measure bigness via measurements
of some other, natural measures of men, such as height, weight, volume, shoulder
width, etc etc. It is possible that there is no unambiguous choice of those
natural measures which will never contradict each other and provide a
single-valued measure of bigness. It is possible that any two natural measures
we choose would in some case, even if not always, provide mutually exclusive
answers as to which man is bigger (i.e. a man X is taller than man Y, but has a
smaller weight than that of Y, or has wider shoulders, but shorter feet,
etc).
Remember though that our goal was to analyze the male populations of
various countries rather than to compare "bigness" of any two individual men.
Therefore, we have to choose certain quantities which would characterize
"bigness" of men in statistical sense. We have here a plenty of choices. For
example, we can choose mean weight of men as the aggregate measure of their
bigness in each country. Or we may choose a cumulative measure of men's bigness
as follows. Exclude all men whose weight is below, say 60 pounds, as well as all
men whose weight is over 250 pounds. Exclude all men younger than 13, as
well as all men older than 85. For each of the rest of men, calculate a function
which is as follows: (square "weight multiplied by some coefficient measured in
m/kg") plus (square height) plus (square shoulder width) plus (square foot
size). Call this function IB, which stands for "individual bigness." By
constructing such function, we hope to include into "bigness" several natural
characteristics, which would level off discrepancies between, say weight and
height, or between shoulder width and foot size, etc). Choosing a
combination of several natural characteristics instead of using only one of them
seems to be a reasonable way to measure "bigness" in a consistent way.
However, the ultimate judgment of whether our IB function reflects an objective
characteristic of male population can be done only when the results of
measurements are obtained and analyzed in regard to their consistency.
Now we have to choose a cumulative statistical measure of "bigness" for
the entire male population of a country. It can be done in many different
ways. For example, sum up all the IB's obtained for men in a
country, and call the sum P1. To have more than one measure, choose
one more aggregate criterion of bigness, for example as the product of all
IB's, and call it P2. Then, introduce two more measures of
bigness, calculated by the same formulas, but applied to little different,
slightly truncated lists of men. Namely, exclude from calculation all men who
have lost a limb to an accident or to a surgery. A cumulative measure of
"bigness," calculated the same way as P1, but applied to the
described truncated list of men, will be denoted P3 , while a measure
calculated exactly as P2 but for the truncated list of men, will be
denoted P4.
Naturally, since the numbers of men in each country are very large, it
is impractical to measure heights, weights, etc, of all men. Therefore we will
choose a reasonably big sampling, say, consisting of 10000 men in each country,
and measure all four P for them.
When all P are found for a set consisting, say, of 150 countries, we
arrange the obtained values of each of four P's in ascending orders. The values
of each P for Ourcountry occupy certain places on the four "ladders" of
P. If a certain country has the minimum value of a P among all the countries
studied, we assign to that country rank 1. The country whose P is the next
smallest, is assigned rank of 2, etc. Let us assume Ourcountry has rank
r on the ladder of ranks created as described. We peruse the tables of
ranks and notice that using four P's resulted in four different ranks of
Ourcountry. For example, in the ladder of ranks obtained by using
P1, Ourcountry has rank r1, while on the
ladder of ranks obtained by using P2, the rank of Ourcountry
is r2 , and
r1>r2.. At the same time, some
country XYZ has a rank below r1 in the list obtained by
using P1 but it has a rank higher than r2 on the
list obtained by using P2. Then in which country the men are bigger,
in Ourcountry or in XYZ? If we rely on P1, we are proud to
conclude that the men in Ourcountry are bigger than in XYZ. However, if
we rely on P2, our national pride is wounded by the conclusion that
the men in XYZ are bigger than in Ourcountry.
Conclusion? One of the following conclusions must be made: 1)There is no
such single-valued property of male population as "bigness;" Or 2) the measures
such as height, weight, shoulder width, and foot size, are not good choices to
measure bigness even if "bigness" could be defined in a logically
uncontroversial way; Or 3)Our technique to measure some of those four
characteristics was faulty; or 4) Our formula for IB was unnatural and did not
reflect "bigness," even if bigness is a meaningful concept; or 5) At least some
of our cumulative measures P1-P4 have been meaningless
combinations of properties. In other words we will have to conclude that
our experiment was a failure.
The above example was as close to what happened in WRR's study as it was
practically possible to make. The differences between the above example with
"bigness" of men and WRR's measurement of "proximity" are in inconsequential
details only. It illustrates the statement that WRR's results are
unreliable.
Example 4. The case of a non-existence of a cumulative
criterion
Let us imagine that we want to compare, using a certain integral quantity,
the religious affiliations of the populations of two countries. A good example
would be Yugoslavia before its breakup, vs, say Italy. Would it be
possible to define a logically consistent cumulative measure reflecting
religious affiliations of those countries' populations? I believe such an
aggregate characteristic does not exist. Nevertheless, imagine that an attempt
has been made to define such a quantity. Imagine further that a survey has been
conducted on samplings of population in each country, which included
representatives of Catholics, Orthodox Christians, and Moslems. Each individual
was assigned a quantitative value depending on his/her religion. For example,
each Catholic would be assigned a value of x, each Orthodox Christian a value of
y, and each Moslem a value of z. After all participants in the survey had been
accounted for, some cumulative measure P would be calculated, for example a sum
of individual "values." Let us assume it has been found that the cumulative
quantity for Yugoslavia was P1, and for Italy it was some
P2. What is the informative significance of those numbers? None!
These numbers do not shed light on anything of consequence and do not add to any
knowledge about the countries in question. One may choose any number of other
ways to assign "values" to individuals in regard to their religion, but there is
no way to make sense of any integral quantity obtained on the base of somehow
combining those numbers. The reason for that is obviously the non-existence of a
logically consistent single-valued quantity characterizing religious affiliation
of people. In the above example the absence of such a cumulative measure was
obvious. In some other cases it may be not self-evident, but can often be
possible. When the existence of a natural cumulative measure of a phenomenon is
not obvious, it may be postulated, but the postulate's validity must be verified
by observing the behavior of the postulated cumulative measure.
Example 5. Distributions vs cumulative
criteria
The following example can clarify the cognitive power of distributions as
compared with cumulative measures. Imagine that we want to compare populations
of two countries in regard to the men's height. Let us say that in one of those
countries there are two ethnic groups. Men in one of those ethnic groups are
typically very tall, while men in the second ethnic group are typically rather
short. In the other country there is only one ethnic group. In both countries we
choose representative groups of men, each consisting of, say, 10000 men, and we
take care to choose the participants in the survey in an unbiased way, i.e.
including into the sampling men from all regions of the country, from all age
groups, professions, etc. Assume we have found that the mean height of a man is
about the same in both counties. What is the informative value of that result?
Obviously, rather than shedding light on the question asked, this result
actually hides the factual situation. The integral quantity chosen for the
evaluation of the men's height, instead of illuminating the problem, provides
for a misleading and meaningless conclusion that men in both countries are of
about the same height.
The situation changes if instead of a cumulative measure we resort to
studying distributions. Seeing the distribution curves of the men over their
height, we discover that in one country there are two distinctive groups of men,
one short and the other tall; we see what are relative strengths of these two
groups; we see also that in the other country men all belong to one group in
regard to their height, and, for example, that typically the men in the second
country are of an height that is between the typical heights of men in the
"tall" and in the "short" groups of the first country, etc. Distributions
provide for a manifold material which is much more informative than the
aggregate measures can ever be, not to mention that distributions always tell
the truth while cumulative quantities often hide it.
: When all the above statements have
been made, one more question still remains to be answered. It
is the question why the "ranks" calculated by WRR have happened to be as small
as they are for the non-permuted data list as compared with its multiple
permutations. This question is quite apart from the topic of the discussion in
this article, which dealt with establishing the validity of the criteria
P1, P2. P3, and P4 as objective properties of the texts. The question
in regard to the small values of ranks found by WRR for the text of the Book of
Genesis has to be answered regardless of the validity of the ranks in question
as objective measures of the text's properties. It poses a challenge to one's
curiosity. I believe, the "riddle" about small "ranks" of the non-permuted list,
which were observed only for the text of Genesis, but not for other texts, has
been quite convincingly solved in a number of publications, for example in
10]. It was demonstrated that a slight modification of the data list can
cause drastic variations in the measured "ranks." In one such case [10] where
WRR have claimed a rank of 1 for the non-permuted list, a slight modification,
by the author of [10] of the data list, where the modified list appeared to be
at least as good as the one used by WRR, and even a more reliable one, the rank
of the non-permuted list changed from 1 to 289000. I believe these facts
eliminate any need for a further search for explanations of the WRR's
extraordinary reports.
1. M. Perakh, posted in this web site (Some Bible-code related experiments and discussions)
2. M. Perakh, posted in this web site ( Do the ELS in the Bible indeed spell what they have been claimed to spell?).
3. M. Perakh, posted on this web site ( Some remarks in regard to D. Witztum's writings concerning the "code" in the book of Genesis).
4. D. Witztum, E. Rips, Y. Rosenberg.
Statistical Science, 9, No 3, 429-438, 1994
5. D. Witztum, E. Rips, Y. Rosenberg. This
article is posted on Brendan McKay's website.
6. D. Witztum, E. Rips, Y. Rosenberg. Preprint
accompanying a presentation to the Israeli Academy of Sciences in 1966 (English
translation). It is posted (without appendix) on ( Mark Perakh's website).
7. A. M. Hasofer. This article is posted on
this web site (A statistical critique of Witztum et al paper).
8. R.J. Larsen, M.L. Marx. An Introduction to
Mathematical Statistics and its Applications. Prentice-Hall, 1986
9. D. Bar-Natan, B. McKay, S. Sternberg. This paper
is posted on Brendan McKay's website
10. B. McKay. This paper is posted on Brendan McKay's website.
11. M. Perakh, Surface Technology, 4,
538-564, 1976.
|
 |